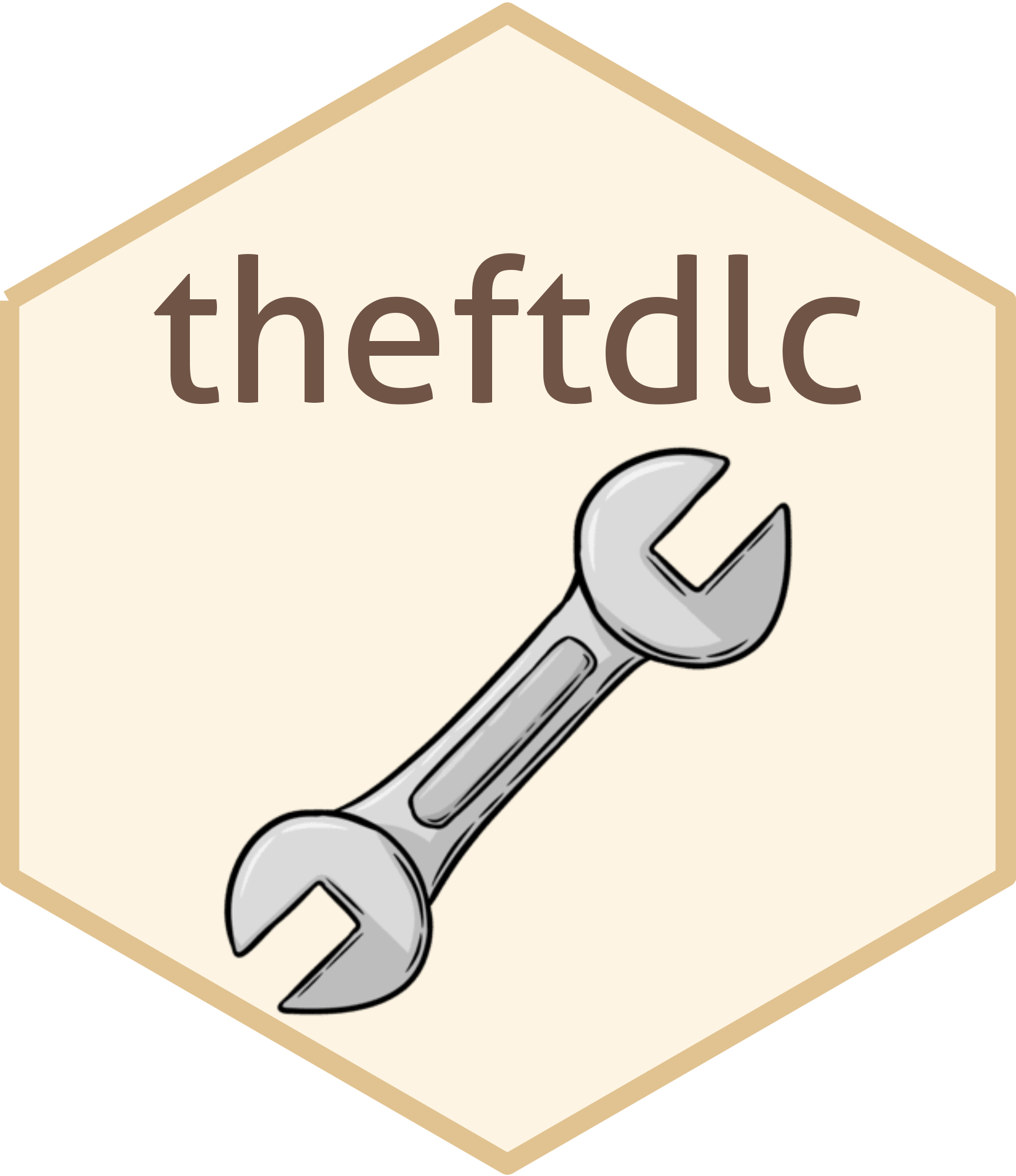
Calculate interval summaries with a measure of central tendency of classification results
Source:R/interval.R
interval.Rd
Calculate interval summaries with a measure of central tendency of classification results
Usage
interval(
data,
metric = c("accuracy", "precision", "recall", "f1"),
by_set = TRUE,
type = c("sd", "qt", "quantile"),
interval = NULL,
model_type = c("main", "null")
)
calculate_interval(
data,
metric = c("accuracy", "precision", "recall", "f1"),
by_set = TRUE,
type = c("sd", "qt", "quantile"),
interval = NULL,
model_type = c("main", "null")
)
Arguments
- data
list
object containing the classification outputs produce bytsfeature_classifier
- metric
character
denoting the classification performance metric to calculate intervals for. Can be one of"accuracy"
,"precision"
,"recall"
,"f1"
. Defaults to"accuracy"
- by_set
Boolean
specifying whether to compute intervals for each feature set. Defaults toTRUE
. IfFALSE
, the function will instead calculate intervals for each feature- type
character
denoting whether to calculate a +/- SD interval with"sd"
, confidence interval based off the t-distribution with"qt"
, or based on a quantile with"quantile"
. Defaults to"sd"
- interval
numeric
scalar denoting the width of the interval to calculate. Defaults to1
iftype = "sd"
to produce a +/- 1 SD interval. Defaults to0.95
iftype = "qt"
ortype = "quantile"
for a 95 per cent interval- model_type
character
denoting whether to calculate intervals for main models with"main"
or null models with"null"
if theuse_null
argument when usingtsfeature_classifier
wasuse_null = TRUE
. Defaults to"main"
Examples
library(theft)
features <- theft::calculate_features(theft::simData,
group_var = "process",
feature_set = NULL,
features = list("mean" = mean, "sd" = sd))
#> No IDs removed. All value vectors good for feature extraction.
#> Running computations for user-supplied features...
#>
#> Calculations completed for user-supplied features.
classifiers <- classify(features,
by_set = FALSE,
n_resamples = 3)
#> Only one set of 'catch22', 'feasts', 'tsfeatures', or 'Kats' with potential duplicates is in your feature data. Exiting and returning original input data.
#> Fitting model 1/6
#> Fitting model 2/6
#> Fitting model 3/6
#> Fitting model 4/6
#> Fitting model 5/6
#> Fitting model 6/6
interval(classifiers,
by_set = FALSE,
type = "sd",
interval = 1)
#> names .mean .lower .upper
#> 1 User_mean 0.1851852 0.1723552 0.1980152
#> 2 User_sd 0.6148148 0.5634948 0.6661348